DATA SCIENCE IN FINANCE
TUESDAYS & THURSDAYS
7 PM BST
5 SEP 2024 - 22 OCT 2024
DURATION:
7 WEEKS
TUESDAYS & THURSDAYS
7 PM BST
Get proficient in financial data science. Deep dive into datasets, and pick up the skills, tools and processes you need to excel in this rapidly evolving industry.
Explore Data Science in Finance with Andrea Augusto Baroni, Head of Data Science Platform at Barclays, for a Data Science in Finance course designed to help you excel with financial data.
WHO THIS COURSE IS FOR
-
YOU ARE AN ANALYST IN THE FINANCIAL SECTOR
Improve your proficiency in financial data science and specialise your skill set. Learn advanced analytics and machine learning techniques for risk assessment and forecasting. Get hands on with building real-world applications including fraud detection systems. Raise your awareness of ethical considerations and regulations in AI deployment in finance.
-
YOU ARE A DATA ANALYST
Take the next step in your career as a data analyst and start to advance in the finance industry. Equip yourself with the practical skills you’ll need, including those to truly set you apart such as Python proficiency and ethical awareness. Embrace the networking opportunities as you learn, live online with an expert instructor.
-
YOU ARE A SCIENTIST, ECONOMIST OR ENGINEER
Ready to pivot from your current role into the financial field? Get the industry specific knowledge you’ll need and the opportunity to gain practical, hands-on experience with projects and workshops. Facilitate a smooth transition for yourself and set yourself up for success.
The UK finance industry is worth over £278 billion.
Keep ahead of the competition and get hands-on experience working with financial data sets. Learn the skills you need to move into a data science role within the world of finance. Enhance your knowledge of machine learning techniques and advanced analytics.
Live, online lessons will be delivered alongside 5 workshops, and 8 demos using Python. Complete a final project centred around building an industry-grade model. Receive 1:1 mock interview practice, alongside the chance to network with other finance professionals.
Build an industry-grade model ready for live deployment. You’ll leave the course with an individual project to show future employers - containing a comprehensive report, code base in Python, visualisations and a key presentation.
Learn from one of the major players in this fast and continually evolving industry. In addition to twice weekly lessons with a renowned expert, you’ll be exposed to a guest speaker covering topics around generative AI.
Get practical. Enter a role in the financial industry confident in your ability to put knowledge into practice. You’ll complete 5 workshops and 8 demos, using one case study throughout the course to address common challenges in financial data.
Andrea Augusto Baroni
LinkedIn Profile- Head of Data Science Platform at Barclays
- Over six years of experience driving impactful initiatives through innovative data science, machine learning, and AI solutions in the Banking, Payments and Telecommunications industries.
- Heads a global team of data scientists, leading strategy and delivery of high-impact projects.
- Spearheaded the cloud modernisation and onboarding program across various analytical personas and use cases, focusing on building a next-generation Cloud Data Science platform.
- Advised FTSE 100/250 clients on data-driven growth strategies and consulted on developing analytical and modelling capabilities in a variety of industries.
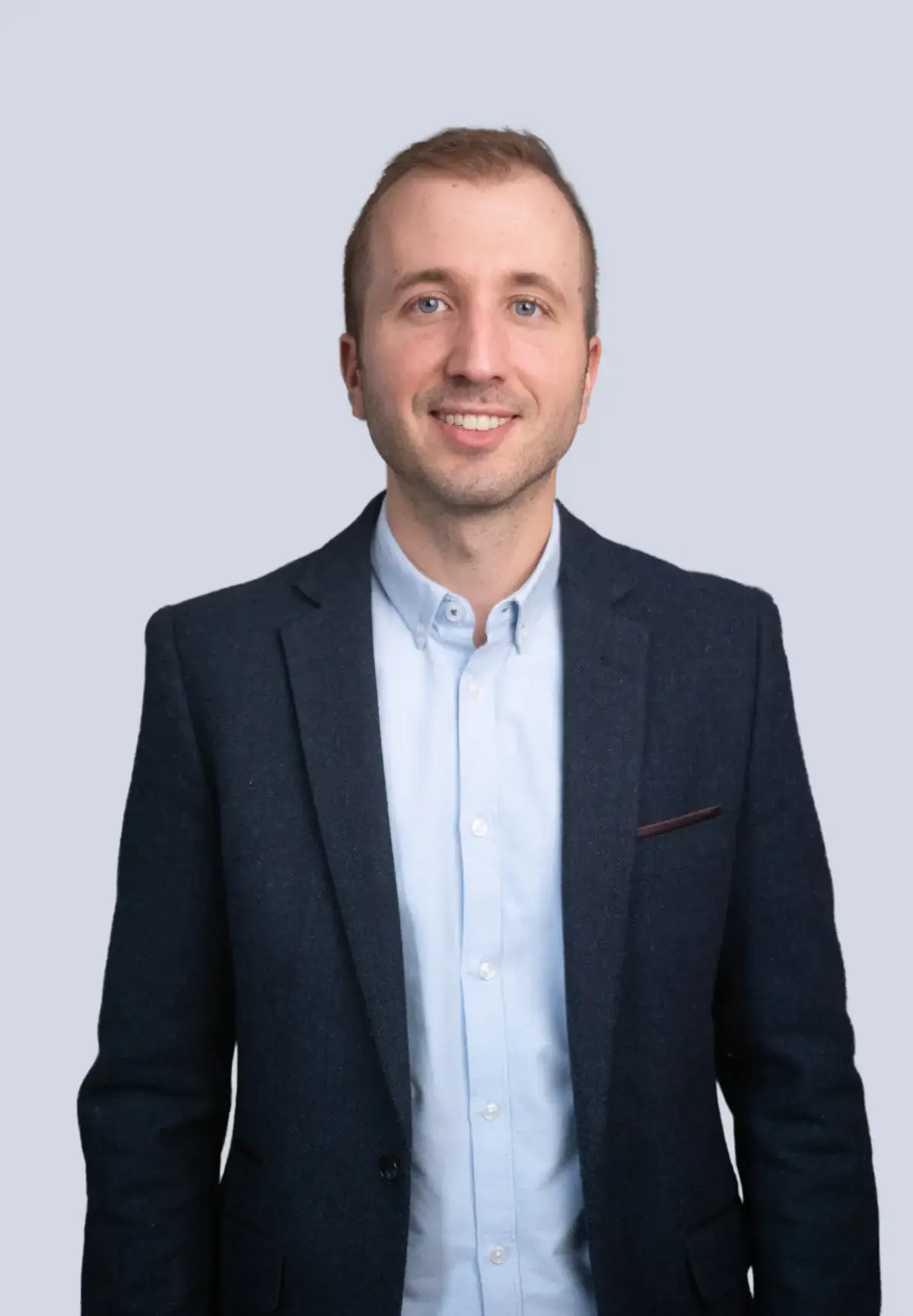
COURSE INTRODUCTION
MODULE 1
Getting Started
Let’s get started! Meet your instructor and discuss the course objectives. Get your Python environment setup, and get any questions you have answered.
- Instructor Introduction
- Course Objectives & Flow
- Python environment setup
- Introduction of project assignment and key dates
- Q & A
Uncover the power of AI and ML in finance. Start to decode key terminology and dive into the Machine Learning workflow.
- What is data science, AI & ML?
- Key applications in financial services
- Key skills for a data scientist or data engineer
- Key terminology and software related to financial services
- Machine Learning pipeline & workflow
MODULE 2
Essentials of Financial Data Processing & Analysis
Learn how to proficiently acquire and clean financial datasets using Python. You’ll cover data sourcing and cleaning techniques as well as addressing common challenges such as imbalanced data.
- Common data sources for data science and AI in finance
- Common data gathering and cleaning techniques and implementation in Python
- Case Study: Addressing common challenges in financial data
- Workshop: Hands-on data cleaning techniques using Python
Get equipped with the skills you need to explore and analyse data in Python. You’ll be able to derive actionable insights through techniques including summary statistics, visualisation and time series analysis.
- Data exploratory analysis and uses in finance
- Feature engineering and its use in data science
- How visualisation can boost your EDA process
- Time series data and how to treat it in EDA
- Demo: Implementation in Python
Assignment #1: Data cleaning and uncovering additional actionable insights as an outcome of EDA.
MODULE 3
Advanced Analytics and AI/ML for Finance Professionals
Get familiar with statistical modelling and gain foundational skills in finance using Python. You’ll cover hypothesis testing, the Central Limit Theorem, and practical applications such as creditworthiness assessment.
- Introduction to statistical modelling and use cases in finance
- How to make inferences and validate business hypotheses with your data
- Workshop: Creditworthiness Assessment
Deep dive into machine learning and artificial intelligence. Discover methods of building and evaluating classification models for risk assessment. Get familiar with ethical considerations, managing bias, and balancing model accuracy with interpretability.
- Introduction to Machine Learning and Artificial Intelligence
- AI & ML algorithms
- Building classification models for risk assessment
- Evaluating and interpreting ML models for risk
- Balancing model accuracy and interpretability
- Common challenges in modelling and techniques to handle them
- Demo: E2E model training and evaluation flow
Assignment #2: Build a baseline model and optimise profitability of a lending book based on credit history.
MODULE 4
Advanced Machine Learning and Deep Learning in Finance
Start your journey to mastering ensemble techniques in machine learning - and understanding their use cases in Finance. Grasp the importance of Model interpretability and explainability frameworks in this specific industry, and get an introduction to hyperparameter tuning.
- Ensemble models in Machine Learning
- Model interpretability and explainability frameworks
- Introduction to Hyperparameter Tuning
- Demo: Implement an ensemble model, performing hyperparameter tuning and compare performance to the baseline model built in session 4
Ready for your introduction to deep learning and neural networks? Focus in on their relevance in finance, and learn about the most popular Python frameworks for implementation.
- Introduction to Deep Learning and Neural Networks
- Understanding how these are used in Finance
- Demo: Popular Python frameworks for neural networks
Assignment #3: Build a challenger model to baseline model built in Assignment #2, using concepts from Session 6 and 7.
MODULE 5
Unsupervised ML Techniques & Time Series Modelling in Finance
Gain the key skills you need to excel in unsupervised learning for finance. You’ll cover outlier detection techniques, strategies for customer segmentation, and dimensionality reduction methods such as principal component analysis.
- Outlier detection: use cases and techniques in financial services
- Clustering and segmentation use cases
- Principal component analysis for dimensionality reduction
- Demo: Implementation in Python
Assignment #4: Segment a credit card customer base into groups for marketing and customer management purposes.
It’s time to master time series modelling in financial services. Start to understand time series data, decomposition techniques and get familiar with foundational forecasting methods.
- Time series data and use cases in finance
- Decomposing time series data into its key components
- Forecasting methods and model families
- Demo: Implementation in Python
Assignment #5 (optional): Forecasting exercise
MODULE 6
Generative AI and building Python apps
Delve deep into Generative AI and Large Language Models. You’ll gain a critical understanding of their functionality and applications in financial services. Explore adoption patterns, risks and opportunities, with a practical demonstration on building a customer service chatbot.
- Introduction to Generative AI and Large Language Models
- Adoption patterns in financial services, risks and opportunities
- Demo: build a customer services chatbot
- Guest speaker
Grasp the opportunity to build a real-time fraud detection app in Python. You’ll get a detailed introduction to app development, a hands-on workshop, and considerations on scalability and design.
- Introduction to building apps in Python
- Workshop: Build a fraud detection app
- Considerations on app scalability and design
Assignment #6 (optional): Build your own app
MODULE 7
From an Idea to Production: How to Deploy Data Science Projects in Finance and Collaboration Best Practices
Get to know ML system design, the ML Ops lifecycle and model governance. Look critically at insights into ethical considerations, data privacy, security concerns, and regulatory compliance in AI deployment within the finance sector.
- ML system design and architecture
- ML Ops lifecycle and model governance
- Ethical considerations of AI in finance
- Data privacy & security concerns
- Regulations and compliance
Assignment #7 (optional): Write architecture diagram for your modelling solution
Want to learn to deliver data science projects effectively? Dive deeper into how to measure success, apply coding best practices, collaborate efficiently, and stay updated on cloud technologies and industry trends.
- Effective data science project delivery
- Measuring success and ROI
- Workshop: Define success for your data science initiative
- Coding and software development best practices
- Effective collaboration
- The rise of cloud and trends in data science industry
Assignment #8: Push code to git
MODULE 8
Career development tips and outlook
Plot your career path in data science within finance with a strategic planning session designed to start your ascent. Discuss key trends in AI as well as the future of finance. Get guidance on how to prepare your CV and how to stand out at interviews.
- Career paths in Data science in finance
- Key trends and AI & finance future
- CV preparation & interview guidance
- Course wrap up
Final Project:
- A comprehensive report documenting the entire process; from data cleaning to model development and deployment. Give clear explanations of methodologies used and architecture considerations
- Code base in Python
- Visualisations communicating key insights derived from the financial dataset
- A presentation where you will effectively communicate findings and decisions to simulate real-world scenarios
What our students say
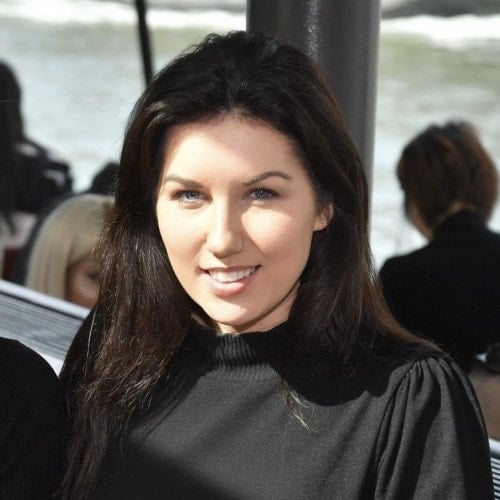
MARKETING DIRECTOR
"It was such a great experience, well worth the course fee which I invested personally - I've learnt so much and feel much more confident in my role.."
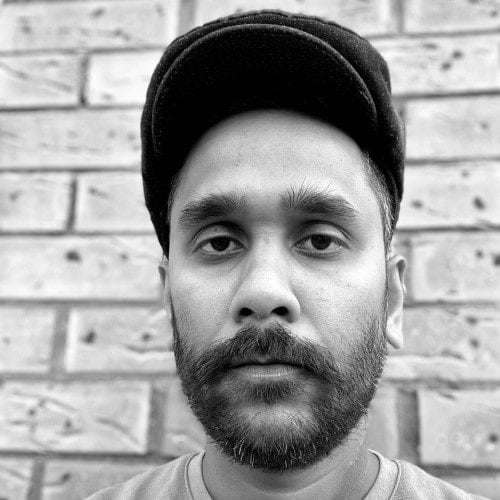
"The course at ELVTR was a great investment in my career. The materials are top-notch, and the instructors provided excellent support."
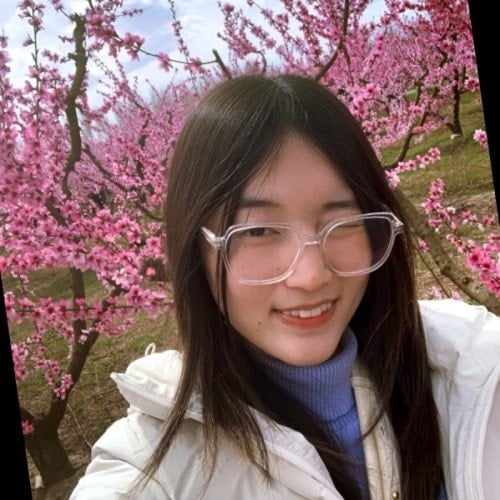
"The knowledge. The teacher is very experienced. He is able to answer our questions in depth, and takes the time to do so."
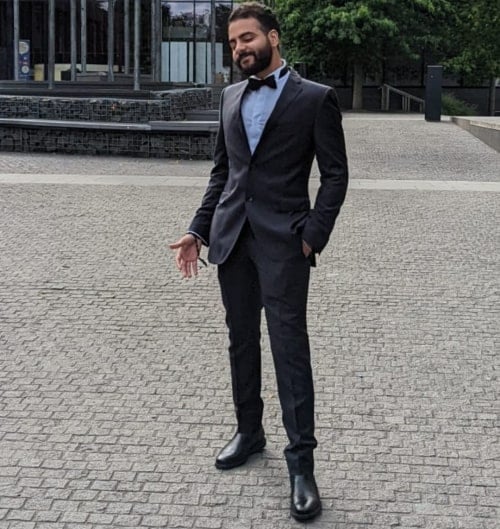
"The course was very helpful in helping me expand my design toolkits by gaining a wealth of new knowledge and inside expertise to stay at the forefront in an age of rapidly developing technological and software advancements."
Fill in the form to start your ELVTR journey.
We will contact you to clarify all the details.